Emerging Dangers: Generative AI’s Risks on Automation Security
Where automation is concerned, generative AI (artificial intelligence) is both a sign of progress and another threat that could expose damaging security vulnerabilities. As organizations embrace generative AI tools like Microsoft Copilot to simplify and scale automation, a new dimension of risk is introduced that must be mitigated.
This article uncovers the security risks and vulnerabilities that generative AI poses to automation practices and what actionable steps can be taken to manage those risks.
Generative AI in Automation
Generative AI and its synonymous tools like ChatGPT continue its rapid ascent to the top of the hype cycle. We’ve written extensively on how it’s poised to revolutionize automation and even discussed it in a recent webinar we hosted.
The models that power generative AI make it easier for the average business user to deliver automations. For example, Microsoft Copilot offers a “describe it to design it” feature that enables users to simply describe an automation, and Copilot will construct and develop it. According to Microsoft, this approach has already been proven to halve development time and exponentially increase the deployment of automations.
As a result, governing and ensuring automations are high quality and secure will become increasingly difficult, heightening the risk of security vulnerabilities and incidents.
The Security Threats Generative AI Poses to Automation
While generative AI is certainly taking automation to new heights and unlocking development for the average business user, it’s not without its security concerns, which include:
#1 – Negligent Attention to Security
Whether we’re talking about RPA (robotic process automation) or something beyond, automation is essentially software development at its core. Almost all organizations have an SDLC (software delivery lifecycle) process that governs how software is developed and delivered. Security is a significant part of that process.
Highly skilled technical developers think about security as they’re developing automations. A QA (quality assurance) process also provides another layer of testing to identify security vulnerabilities.
Average businesspeople designing and delivering automations with the help of AI will not devote the same attention to security and therefore leave room for vulnerabilities that could lead to damaging incidents.
#2 – Lackluster Credential Management
Another way AI can impact automation security is by diminishing secure credential management. Automated processes interact with numerous systems and applications that make up your enterprise architecture and handle a large amount of sensitive data. Because of that, the credentials that automations require to carry out their tasks must be managed and protected.
With AI's help, the average business user will not show the same diligence as a seasoned developer to mitigate the security risk here.
#3 - Increased Risk of Non-Compliance
Whether your organization operates in a highly regulated industry or not, all companies that handle sensitive data must comply with data privacy regulations like GDPR, HIPAA, or CCPA.
Normal SDLC processes have compliance checks built in. The same can’t be said for the average business user developing and delivering automations with the help of AI, significantly increasing the risk of non-compliance and the penalties and potential hefty fines that come along with it.
How to Mitigate the Threats Generative AI Poses to Automation
To minimize the threats that generative AI poses to automation, organizations can adopt the following strategies:
#1 – A Commitment to Documentation
Documenting all deployed automations doesn’t just help with troubleshooting and maintenance should errors occur and need to be investigated; they also help reinforce security.
Implementing a strict policy to draft a process design document (PDD) or, ideally, have one automatically generated with technology ensures that automation leaders can maintain holistic visibility on their automation estate and investigate security vulnerabilities at will, particularly with automations designed and delivered with generative AI assistance.
#2 – Robust Security Testing
Security testing is typically part of every company’s SDLC. The same can’t be said with automations the average business user delivers.
Performing manual security tests with all automations produced with generative AI may be unfeasible due to the required effort. The safest and most secure bet is implementing a solution that could run automatic testing on all automations, regardless of who designed them.
Conclusion
As generative AI continues to rapidly creep into the world of automation, the security implications and concerns it’s raising cannot be ignored. Negligence, lackluster credential management, and increased risk of non-compliance are just the tip of the iceberg.
As more business users start to produce and deploy simple automations into production, a proactive and robust approach to automation security – like the one we outline in the 8 Steps to Build a Robust Security Framework for Automation – might not be enough. Instead, organizations will need to augment their automation security practices with purpose-built technology that can automatically run security checks and generate documentation to keep their automations as secure as possible.
Share this
Recent Stories
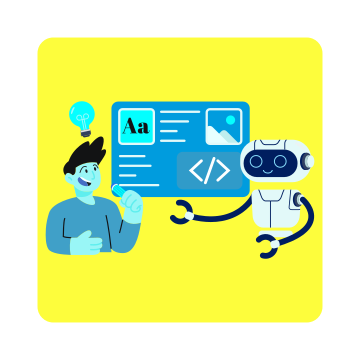
The Risks of AI in RPA and How to Avoid Them
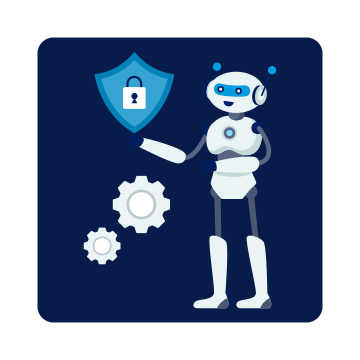
The 5 Pillars of Automation Security
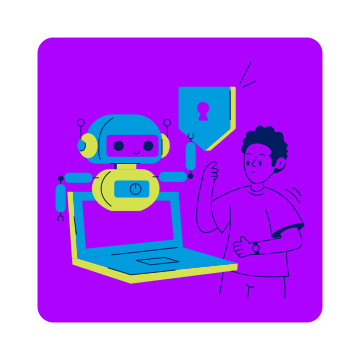