How to Manage Automation Sprawl
Robotic process automation (RPA) is continually changing. Like most enterprise software categories, the next iteration involves including artificial intelligence (AI).
Specifically, AI-assisted RPA design and delivery, akin to what Microsoft Copilot offers, appears to be the next big thing, quickly making its way to the apex of the hype cycle.
The idea of accelerated, democratized RPA delivery that doesn’t depend so heavily on IT resources sounds promising. Business users being able to design and deliver automated processes without the traditional technical expertise that was needed to achieve scale is great. Still, it doesn’t mean that there aren’t disadvantages or risks to assisted automation.
The concern is that open and unabated RPA delivery could lead to what’s being referred to as automation sprawl; where organizations lose control of their automation practice and become riddled with ineffective, error-prone bots that inflate costs instead of reducing them while gaining little in terms of productivity and efficiency. To help RPA leaders realize the benefits of a rapidly changing automation landscape, this article explains what automation sprawl is and how organizations can mitigate the risks associated with AI-assisted RPA design and delivery.
What is Automation Sprawl?
Automation sprawl is a potential by-product of AI-assisted RPA design and delivery. Because revolutionary solutions like Microsoft Copilot greatly simplify automation delivery for the average business user, the concern is that organizations will no longer be able to vigilantly standardize, control, and oversee automation implementation to ensure a high standard of quality.
The ultimate worry is that assisted automation implementation will resemble the Wild West, and quality will suffer. RPA practices will be bogged down by outages, errors, and inefficient processes that lead to inflated costs and eat away at the returns that automation promises and delivers.
That’s at a high level. AI-assisted RPA design and delivery presents different challenges when digging deeper.
Automation Sprawl: The Risks of Simplified, Unfiltered RPA Expansion
Without the right diligent and robust controls, the democratization of AI-assisted RPA poses the following complexities and challenges:
- Quality and Efficiency: The surge in simplified automation implementation might not always lead to high-quality production. Without the right standards and mechanisms to enforce those standards, poorly designed processes can become inefficient, requiring more maintenance, compromising, and muting any intended productivity gains.
- Security Vulnerabilities: An expansive, loosely governed automation landscape brought on by democratized automation application could also introduce significant security risks, making organizations more susceptible to data breaches and cyber threats. This can be a major detractor, particularly for organizations that operate in highly regulated industries.
- Governance Challenges: Maintaining oversight of an ever-growing automation estate becomes increasingly tricky when controls on RPA delivery are removed, risking the loss of control and visibility over deployed automations. Organizations already struggle with understanding what they have in their RPA estates. With automation design and delivery becoming even more accessible and less controlled, this pain point has the potential to become worse.
How to Mitigate the Risks of Automation Sprawl
Addressing the challenges of AI-assisted RPA design and delivery requires a concerted effort. Greater emphasis needs to be applied to implementing robust governance models and measures with a commitment to digital documentation, as explained in the following:
Enhanced Governance
A robust governance framework is essential for ensuring that the development and deployment of automations – especially where business users leverage AI-assisted RPA design and delivery – adhere to best practices, standards, and processes that safeguard quality, efficiency, and security.
Such a framework and governance model should include clear guidelines and oversight protocols for all stakeholders involved in RPA initiatives, ensuring accountability and consistency across the board.
Where governance can be augmented with continuous testing and code-checking solutions – like any CICD (continuous integration/continuous development) model – such opportunities should always be leveraged to reinforce governance and a high standard of quality.
A Commitment to Digital Documentation
Maintaining a digital record is paramount in an environment where assisted automations are created at an exponential rate.
Documenting key information such as each automation's purpose, creation date, creator, and other relevant details ensures that organizations have a clear and accurate understanding of their automation estate—both in terms of overall size and function that delineates what each automation does to prevent pain-staking mysteries and investigations in the future.
This documentation is vital for governance and instrumental in quickly addressing any issues or errors that may arise.
The Path Forward
As AI-assisted RPA design and delivery redefines the landscape of automation, the emphasis on implementing effective governance and documentation strategies cannot be overstated.
While the benefits of AI becoming more and more baked into RPA are significant, ensuring the quality and security of automated processes is critical. By adopting a proactive approach to governance and documentation, organizations can harness the full potential of simplified and democratized RPA implementation, driving efficiency and innovation while mitigating the risks associated with automation sprawl.
The journey towards AI-enhanced RPA, currently exemplified by Microsoft Copilot, symbolizes a significant leap forward in the quest for operational excellence, efficiency, and cost reduction. As we navigate this new frontier, the balance between innovation and control will be vital to realizing the transformative potential of AI in assisted automation.
Share this
Recent Stories
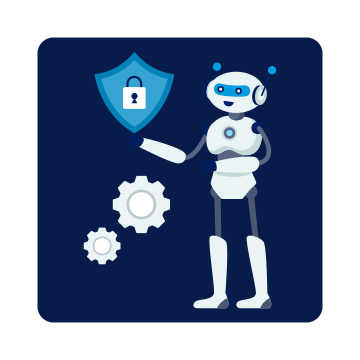
The 5 Pillars of Automation Security
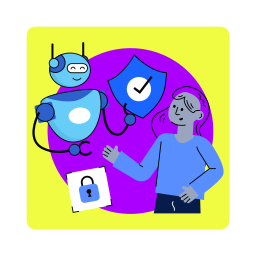
8 Steps to Build a Robust Security Framework for Automation
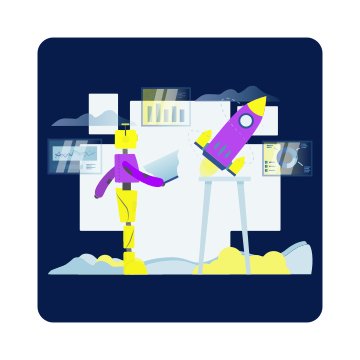